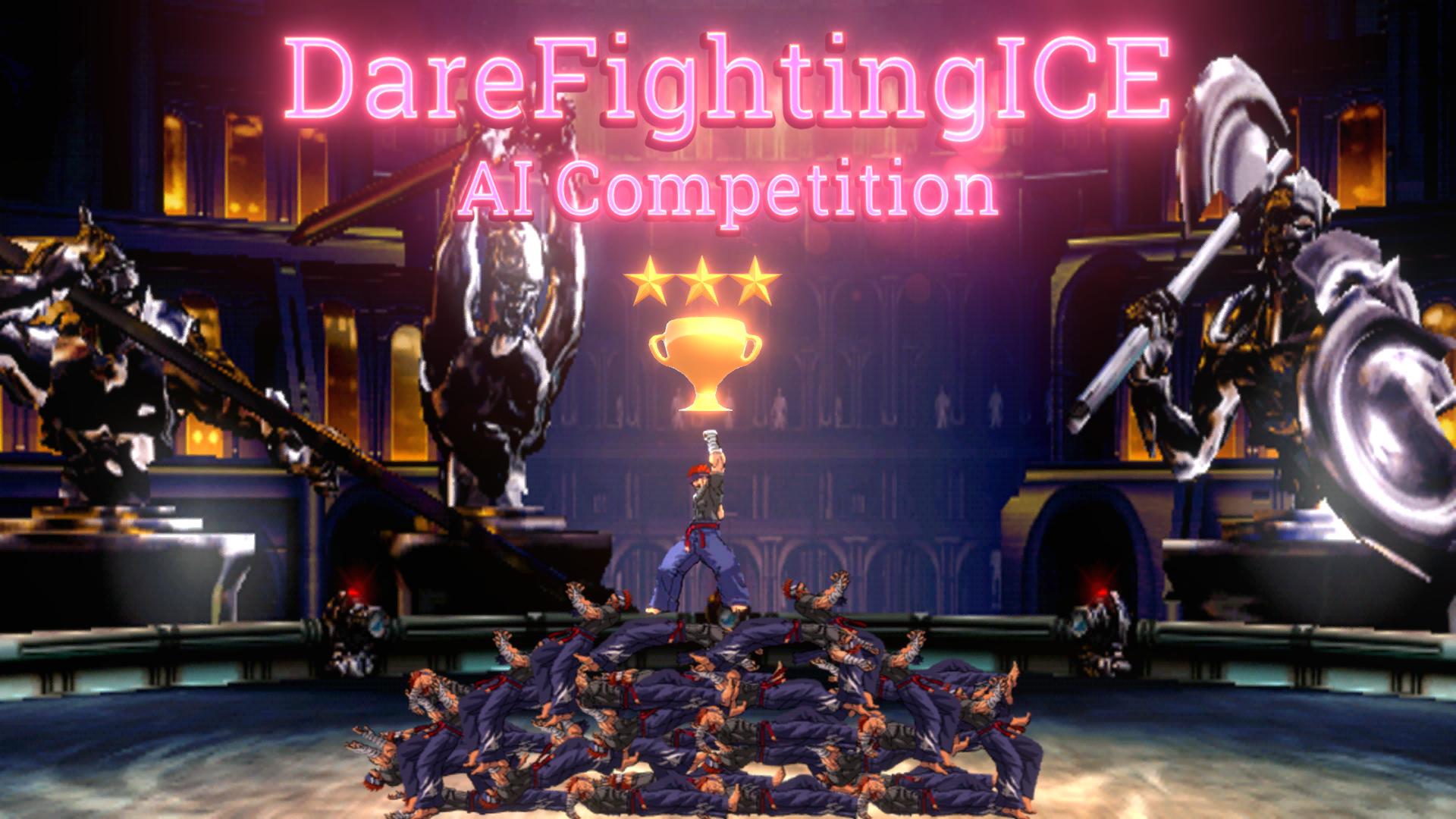
Introduction
Sound plays a crucial role in video games . Various types of sound effects not only enhance players’ in-game experience but also improve their performance. Recent research has shown that, in addition to human players, Artificial Intelligence (AI) players can also benefit from sound, achieving remarkable results. Sound serves as an additional input modality to improve performance of the AI agents. These studies show the potential of research on utilizing sound in video games for AI players.This competition introduces a novel challenge, encouraging participants to develop and submit AI agents capable of interpreting and leveraging sound for gameplay tasks on the DareFightingICE platform.
This competition aims to inspire innovative approaches to sound-based AI solutions in gaming contexts. An AI interface is provided for the participants to develop their own AI. Additionally, we also offer our sample Deep Reinforment Learning Blind AI source code as a reference.
An effective blind agent is crucial in DareFightingICE for several reasons:
It helps promote enhancements in in-game sound design, as the sound design must be informative for the blind agent to understand the current situation in the game, thereby enhancing the in-game sound experience.
It drives advancements in audio processing techniques, as video games often feature a variety of sound cues that require effective processing. This is particularly important in fighting games, where the AI must understand the game environment solely through real-time sound cues.
The winner AI, if trainable, will be used as the opponent agent for the following year’s competition and will be used to evaluate submissions in the subsequent Sound Design Competition. The Sound Design Competition tasks participants with submitting sound designs specifically for the DareFightingICE platform. Consequently, the stronger the blind agent, the more it contributes to improving sound design in DareFightingICE.
Since our competition focuses on game-playing AI, we believe that it aligns seamlessly with the scope of CoG. To the best of our knowledge, this is the first competition dedicated to game-playing AI agents that rely solely on sound inputs.
From an academic perspective, several studies have utilized DareFightingICE, including our sample Deep Reinforcement Learning Blind AI, as a foundation for their research. This demonstrates the platform’s relevance and potential, reinforcing our confidence that this competition will attract significant attention from the research community.
Logistics
Entries to the competition will be submitted via google form which is available on our GitHub. The competition results will be announced on the competition web page. In our competition, there are two leagues that are describe below:
The Standard League considers the winner of a round as the one with the HP above zero at the time its opponent’s HPhas reached zero. Both AIs will be given the initial HP of 400. The league for a given character type is conducted in a round-robin fashion with two games for any pair of entry AIs switching P1 and P2. The AI with highest number of winning rounds becomes the league winner; If necessary, remaining HPs are used for breaking ties. In this league, our weakened sample MctsAi with limited to 23 iterations per frame, played in the non-blind mode or with FrameData, and our sample deep-learning blind AI, played in the blind mode, will also be participating as baseline AIs.
In the Speedrunning League, the league winner of a given character type is the AI with the shortest average time to beat both of our aforementioned sample AIs. For each entry AI, 5 games are conducted with the entry AI being P1 and a sample AI being P2, and another set of 5 games with the entry AI being P2 and a sample AI being P1. Both AIs will be given the initial HP of 400. If a sample AI of interest cannot be beaten in 60s, the beating time of its opponent entry AI is penalized to 70s.
In each of the two leagues (in this order: Zen Standard, Zen Speedrunning), the AIs are ranked according to the number of winning rounds. If ties exists, their total remaining HPs will be used. Once the AIs are ranked in each league, league points are awarded to them according to their positions using the 2018 Formula-1 scoring system. The competition winner is finally decided by the sum of league points across all two leagues. The participants are provided with details and guides on installation and the working of our system in our GitHub page.
Participants
This competition was previously held at the IEEE Conference on Games in 2023 and 2024. Our target participants include students at undergraduate and graduate levels, as well as researchers and developers who are interested in game playing AI.
Previous years’ competitions held at CoG:
Timeline
Midterm deadline: June 7, 2025 (AoE)
Final deadline: August 7, 2025 (AoE)
Result announcement: TBA (at the conference)
Organizers
Van Thai Nguyen is a PhD student from Intelligent Computer Entertainment Laboratory, Ritsumeikan University, Japan. His research interests in Machine Learning Reinforcement Learning, especially game playing AI in DareFightingICE. He has published a work on a reinforcement learning blind AI that uses sound as input to play in DareFightingICE in 2022[5]. He has been a part of the DareFightingICE competition team, which has organized the DareFightingICE competitions at CoG for three years, and he serves as the lead organizer of this competition.
Ibrahim Khan is a PhD student from Intelligent Computer Entertainment Laboratory, Ritsumeikan University, Japan. His research interests in sound design for visually impaired people in DareFightingICE. He has been a part of the DareFightingICE competition team, which has organized the DareFightingICE competitions at CoG for three years>
Chuang Boyu is a master student from Intelligent Computer Entertainment Laboratory, Ritsumeikan University, Japan. His research interests in Machine Learning Reinforcement Learning, especially game playing AI in DareFightingICE. He was part of 2024 DareFightingICE AI Competition.
Shouchen Ye is an undergraduate student from Intelligent Computer Entertainment Laboratory, Ritsumeikan University, Japan. His research interests in Machine Learning Reinforcement Learning, especially game playing AI in DareFightingICE.
Ruck Thawonmas is currently a Full Professor with the College of Information Science and Engineering, Ritsumeikan University, Japan, where he is leading the Intelligent Computer Entertainment Laboratory with more than 40 laboratory graduates currently working in game industry. His current research interest includes games for health and for humanities. He is the Director of the DareFightingICE project.